R and Data Analysis: Open-Access Articles
by Janet Salmons, Ph.D., Research Community Manager for Sage Methodspace
Want to use R for statistical computing? These open-access resources might help!
From the R Foundation
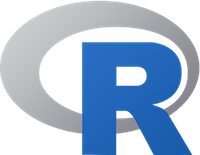
The nonprofit R foundation provides support for the free R software. The R Foundation offers a blog and an open-access peer reviewed journal.
From Sage Journals
Bradley A, James RJE. Web Scraping Using R. Advances in Methods and Practices in Psychological Science. 2019;2(3):264-270. doi:10.1177/2515245919859535
Abstract. The ubiquitous use of the Internet in daily life means that there are now large reservoirs of data that can provide fresh insights into human behavior. One of the key barriers preventing more researchers from utilizing online data is that they do not have the skills to access the data. This Tutorial addresses this gap by providing a practical guide to scraping online data using the popular statistical language R. Web scraping is the process of automatically collecting information from websites. Such information can take the form of numbers, text, images, or videos. This Tutorial shows readers how to download web pages, extract information from those pages, store the extracted information, and do so across multiple pages of a website. A website has been created to assist readers in learning how to web-scrape. This website contains a series of examples that illustrate how to scrape a single web page and how to scrape multiple web pages. The examples are accompanied by videos describing the processes involved and by exercises to help readers increase their knowledge and practice their skills. Example R scripts have been made available at the Open Science Framework.
Brown VA. An Introduction to Linear Mixed-Effects Modeling in R. Advances in Methods and Practices in Psychological Science. 2021;4(1). doi:10.1177/2515245920960351
Abstract. This Tutorial serves as both an approachable theoretical introduction to mixed-effects modeling and a practical introduction to how to implement mixed-effects models in R. The intended audience is researchers who have some basic statistical knowledge, but little or no experience implementing mixed-effects models in R using their own data. In an attempt to increase the accessibility of this Tutorial, I deliberately avoid using mathematical terminology beyond what a student would learn in a standard graduate-level statistics course, but I reference articles and textbooks that provide more detail for interested readers. This Tutorial includes snippets of R code throughout; the data and R script used to build the models described in the text are available via OSF at https://osf.io/v6qag/, so readers can follow along if they wish. The goal of this practical introduction is to provide researchers with the tools they need to begin implementing mixed-effects models in their own research.
Corlatti L. Regression Models, Fantastic Beasts, and Where to Find Them: A Simple Tutorial for Ecologists Using R. Bioinformatics and Biology Insights. 2021;15. doi:10.1177/11779322211051522
Abstract. Regression modeling is a workhorse of statistical ecology that allows to find relationships between a response variable and a set of explanatory variables. Despite being one of the fundamental statistical ideas in ecological curricula, regression modeling can be complex and subtle. This paper is intended as an applied protocol to help students understand the data, select the most appropriate models, verify assumptions, and interpret the output. Basic ecological questions are tackled using data from a fictional series, “Fantastic beasts and where to find them,” with the aim to show how statistical thinking can foster curiosity, creativity and imagination in ecology, from the formulation of hypotheses to the interpretation of results.
Cousineau D, Goulet M-A, Harding B. Summary Plots With Adjusted Error Bars: The superb Framework With an Implementation in R. Advances in Methods and Practices in Psychological Science. 2021;4(3). doi:10.1177/25152459211035109
Abstract. Plotting the data of an experiment allows researchers to illustrate the main results of a study, show effect sizes, compare conditions, and guide interpretations. To achieve all this, it is necessary to show point estimates of the results and their precision using error bars. Often, and potentially unbeknownst to them, researchers use a type of error bars—the confidence intervals—that convey limited information. For instance, confidence intervals do not allow comparing results (a) between groups, (b) between repeated measures, (c) when participants are sampled in clusters, and (d) when the population size is finite. The use of such stand-alone error bars can lead to discrepancies between the plot’s display and the conclusions derived from statistical tests. To overcome this problem, we propose to generalize the precision of the results (the confidence intervals) by adjusting them so that they take into account the experimental design and the sampling methodology. Unfortunately, most software dedicated to statistical analyses do not offer options to adjust error bars. As a solution, we developed an open-access, open-source library for R—superb—that allows users to create summary plots with easily adjusted error bars.
Nordmann E, McAleer P, Toivo W, Paterson H, DeBruine LM. Data Visualization Using R for Researchers Who Do Not Use R. Advances in Methods and Practices in Psychological Science. 2022;5(2). doi:10.1177/25152459221074654
Abstract. In addition to benefiting reproducibility and transparency, one of the advantages of using R is that researchers have a much larger range of fully customizable data visualizations options than are typically available in point-and-click software because of the open-source nature of R. These visualization options not only look attractive but also can increase transparency about the distribution of the underlying data rather than relying on commonly used visualizations of aggregations, such as bar charts of means. In this tutorial, we provide a practical introduction to data visualization using R specifically aimed at researchers who have little to no prior experience of using R. First, we detail the rationale for using R for data visualization and introduce the “grammar of graphics” that underlies data visualization using the ggplot package. The tutorial then walks the reader through how to replicate plots that are commonly available in point-and-click software, such as histograms and box plots, and shows how the code for these “basic” plots can be easily extended to less commonly available options, such as violin box plots. The data set and code used in this tutorial and an interactive version with activity solutions, additional resources, and advanced plotting options are available at https://osf.io/bj83f/.
Rousselet GA, Pernet CR, Wilcox RR. The Percentile Bootstrap: A Primer With Step-by-Step Instructions in R. Advances in Methods and Practices in Psychological Science. 2021;4(1). doi:10.1177/2515245920911881
Abstract. The percentile bootstrap is the Swiss Army knife of statistics: It is a nonparametric method based on data-driven simulations. It can be applied to many statistical problems, as a substitute to standard parametric approaches, or in situations for which parametric methods do not exist. In this Tutorial, we cover R code to implement the percentile bootstrap to make inferences about central tendency (e.g., means and trimmed means) and spread in a one-sample example and in an example comparing two independent groups. For each example, we explain how to derive a bootstrap distribution and how to get a confidence interval and a p value from that distribution. We also demonstrate how to run a simulation to assess the behavior of the bootstrap. For some purposes, such as making inferences about the mean, the bootstrap performs poorly. But for other purposes, it is the only known method that works well over a broad range of situations. More broadly, combining the percentile bootstrap with robust estimators (i.e., estimators that are not overly sensitive to outliers) can help users gain a deeper understanding of their data than they would using conventional methods.
Song QC, Tang C, Wee S. Making Sense of Model Generalizability: A Tutorial on Cross-Validation in R and Shiny. Advances in Methods and Practices in Psychological Science. 2021;4(1). doi:10.1177/2515245920947067
Abstract. Model generalizability describes how well the findings from a sample are applicable to other samples in the population. In this Tutorial, we explain model generalizability through the statistical concept of model overfitting and its outcome (i.e., validity shrinkage in new samples), and we use a Shiny app to simulate and visualize how model generalizability is influenced by three factors: model complexity, sample size, and effect size. We then discuss cross-validation as an approach for evaluating model generalizability and provide guidelines for implementing this approach. To help researchers understand how to apply cross-validation to their own research, we walk through an example, accompanied by step-by-step illustrations in R. This Tutorial is expected to help readers develop the basic knowledge and skills to use cross-validation to evaluate model generalizability in their research and practice.
Learn more in this post, and find Sage books on the topic.
More Methodspace Posts about Data Science and Data Analysis
This blog is the seventh, and penultimate post, in a follow-on to our 2021 “The future of computational social science is Black” series, about a Summer Institute in Computational Social Science organized by Howard University and Mathematica. It continues to bring the power of computational social science to the issues of systemic racism and inequality in America. This marks the third iteration of the successful SICSS model being hosted by a Historically Black College or University.
This blog post is the sixth of eight in a follow-on to our 2021 “The future of computational social science is Black” series, about a Summer Institute in Computational Social Science organized by Howard University and Mathematica. It continues to bring the power of computational social science to the issues of systemic racism and inequality in America. This marks the third iteration of the successful SICSS model being hosted by a Historically Black College or University.
Latanya Sweeney, scholar of technology science, Daniel Paul Professor of the Practice of Government and Technology at the Harvard Kennedy School and in the Harvard Faculty of Arts and Sciences, and director and founder of the Public Interest Tech Lab, delivered the keynote address for SICSS-Howard/Mathematica 2023.
This blog post is the fifth of eight in a follow-on to our 2021 “The future of computational social science is Black” series, about a Summer Institute in Computational Social Science organized by Howard University and Mathematica. It continues to bring the power of computational social science to the issues of systemic racism and inequality in America. This marks the third iteration of the successful SICSS model being hosted by a Historically Black College or University.
This blog post is the fourth of eight in a follow-on to our 2021 “The future of computational social science is Black” series, about a Summer Institute in Computational Social Science organized by Howard University and Mathematica. It continues to bring the power of computational social science to the issues of systemic racism and inequality in America. This marks the third iteration of the successful SICSS model being hosted by a Historically Black College or University.
This blog post is the third of eight in a follow-on to our 2021 “The future of computational social science is Black” series, about a Summer Institute in Computational Social Science organized by Howard University and Mathematica. It continues to bring the power of computational social science to the issues of systemic racism and inequality in America. This marks the third iteration of the successful SICSS model being hosted by a Historically Black College or University.
This blog post is the second of eight in a follow-on to our 2021 “The future of computational social science is Black” series, about a Summer Institute in Computational Social Science organized by Howard University and Mathematica. It continues to bring the power of computational social science to the issues of systemic racism and inequality in America. This marks the third iteration of the successful SICSS model being hosted by a Historically Black College or University.
This blog post is the first of eight in a follow-on to our “The future of computational social science is Black” series, about a Summer Institute in Computational Social Science organized by Howard University and Mathematica. It continues to bring the power of computational social science to the issues of systemic racism and inequality in America. This marks the third iteration of the successful SICSS model being hosted by a Historically Black College or University.
How can you use data science in social science research? Find an interview with the Oxford Internet Institute’s Dr. Bernie Hogan and lots of useful resources in this post.
Daniel Lobo shares four learnings from his unforgettable experience at SICSS-Howard/Mathematica 2022.
UDC Assistant Professor, Andrea Adams, talks about their experience during SICSS-Howard/Mathematica 2022.
Hear from the 2021 SICSS-Howard/Mathematica Excellence in Computational Social Science Research Fund awardees about how the funding helped their projects and their future plans.
At SICSS-Howard/Mathematic 2022 two group projects and five individual projects received the Inaugural Excellence in Computational Social Science Research Award.
At SICSS-H/M 2022, Anthony Wutoh, Yahya Shaikh and Carter Clinton discussed how to bridge the diversity gap in biomedical research and the need for interdisciplinary collaboration.
Rashun Miles discusses his experience returning to SICSS-Howard/Mathematica as an alumni and the larger need for restorative spaces for Black students in every university.
LaVerne H. Council spoke at the Closing Plenary of SICSS/Howard-Mathematica 2022, emphasizing the importance of seeking wisdom and truth through data and fair & effective policymaking.
Paula Moreno, founder of Manos Visibles & first Afro-Colombian woman to serve as Minister of Culture of Colombia, delivered the first SICSS-Howard/Mathematica Motivational Address, focused on the technological challenges and solutions for Africans and people of African descent around the world.
Speakers at SICSS-Howard/Mathematica 2022 explore how change does not affect populations equally, and how the exclusion of underrepresented communities can perpetuate social injustice.
Bite-Sized Lunchtime Talks (BSLT) are a SICSS-Howard/Mathematica site-specific innovation that introduces participants to organizations doing cool things with data. 2022 offered a variety of organizations that proved interesting and inspiring to our participants.
Dr. Safiya Noble speaks about her journey as a critical race scholar, her research on the racist and sexist dynamics of search engines, and the importance of community.
At SICSS-Howard/Mathematica 2022, Shawndra B. Hill, Karen Levy, Brandeis Marshall, and Kyla McMullen emphasized themes of technological determinism, bias, and privacy, and discussed connections to inclusion, equity, and diversity.
The opening plenary of SICSS-Howard/Mathematica 2022 featured a fireside chat with Dr. Anthony K. Wutoh, the Provost of Howard University, and Dr. Amy Yeboah Quarkume, an Associate Professor of Africana Studies, to kick off the event.
The first Summer Institute in Computational Social Science held at a Historically Black College or University, returns to Howard University for its two-day pre-institute, Praxis to Power for graduate students, postdoctoral researchers, and beginning faculty who needed more time to practice computational methods.
In this Methodspace interview Dr. Joel Thurston and Dr. Cesar Montalvo tell us about how data science can be used for social good, and how their program for young scholars is cultivating a next generation of data scientists.
In this Sage Methodspace webinar Dr. Matti Nelimarkka and Dr. Friedolin Merhout discuss strategies for engaging with students and building the skills needed to design, plan, and conduct studies using computational social science methods
Learn how computational social sciences help scholars to renew their research in several directions.
This collection of open-access articles offers multiple perspectives on the use of Big Data and ethical protocols for computational research methods.
Summer Institute in Computational Social Science site sponsored by Howard University and Mathematica (SICSS-Howard/Mathematica) awards individuals and teams for the inaugural Excellence in Computational Social Science Research Fund as a unique and exclusive benefit offered to alumni of the site.
A SICSS-Howard Mathematica 2021 participant shares how he reconnected with others in a meaningful way and grew personally during his virtual SICSS experience.
Paul Decker PhD, president and chief executive officer of Mathematica and nationally recognized expert on policy research, delivered the closing plenary address on Friday, June 25th at SICSS-Howard/Mathematica 2021.
This blog post is the eighth, and final, post in a follow-on to our 2021 “The future of computational social science is Black” series, about a Summer Institute in Computational Social Science organized by Howard University and Mathematica. It continues to bring the power of computational social science to the issues of systemic racism and inequality in America. This marks the third iteration of the successful SICSS model being hosted by a Historically Black College or University.